AI for Responsible Innovation: Mitigating Bias and Ensuring Fairness in AI Development
The applications of AI in the B2B sphere raise questions about bias and fairness. As AI advances, responsible AI development becomes critical to effective integration.
This blog explores responsible AI development and solutions for minimizing bias in B2B AI initiatives.
What is Responsible AI development?
Responsible AI development aims to create ethical, safe, and trustworthy AI systems by tackling biases in data collection, algorithm design, and human interpretation, all while encouraging justice, dependability, and responsibility. Responsible AI development requires recognizing biases and creating AI that promotes fairness and equality.
What is Bias in AI?
Bias in AI refers to systematic errors in decision-making that cause unequal outcomes and can be influenced by data collection, algorithm design, and human interpretation.
Machine learning models can learn and replicate bias patterns, producing unrepresentative results. Mitigation strategies include dataset enhancement, bias-aware algorithms, and user feedback mechanisms.
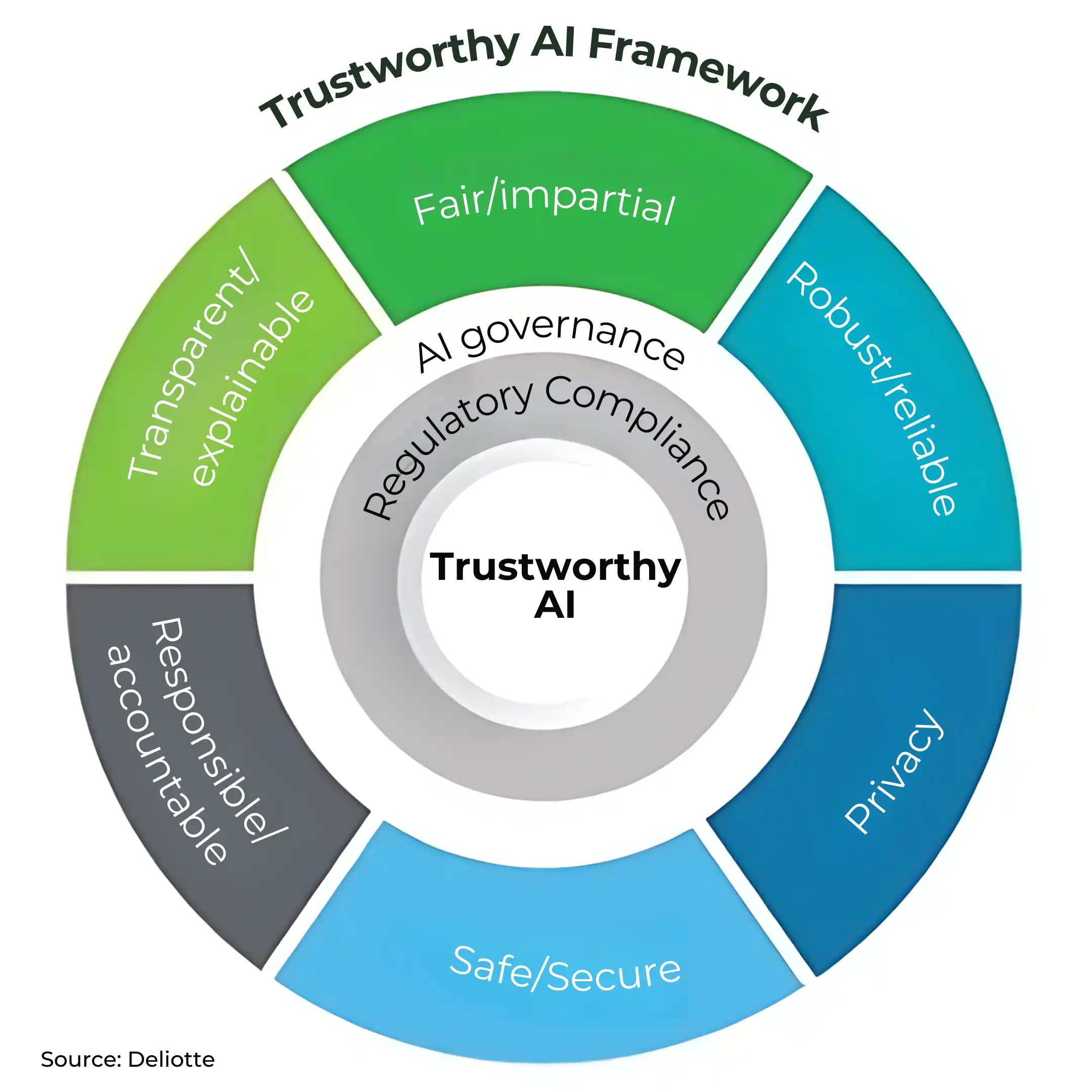
Risks of Bias in AI systems
Biased AI systems can have ethical and social effects, such as favoring certain groups and discriminating against others. They can also promote prejudicial views, resulting in inaccurate evaluations of individuals. Furthermore, inaccurate predictions or recommendations caused by bias can influence key domains such as healthcare and finance, weakening public faith in artificial intelligence.
Biased AI systems can harm enterprises by providing erroneous forecasts and outputs for specific segments of the population. These systems can unfairly allocate opportunities, resources, and information, violate civil liberties, jeopardize individual safety, and fail to offer equal quality service to some people, and have a detrimental influence on a person’s well-being.
These challenges cost businesses money by harming their reputation, consumer trust, and future market prospects.
Microsoft has identified reputational harm or liability from biased AI systems as a danger to its business. AI systems shown to be prejudiced may be abandoned or require extensive adjustments, incurring considerable costs in staff time and other invested resources. Furthermore, biased AI might lead to internal disagreements and employee calls for greater ethical practices. Governments are exploring policies and legislation to address these challenges, and corporations that do not prioritize tackling prejudice in AI may face significant penalties.
The steps to building trustworthy AI in the B2B world include careful development, ensuring AI is responsible and trustworthy, and avoiding bias and unfairness by following specific steps to ensure success.
Guiding Principles for Responsible AI Development
- Fairness and Inclusiveness: AI should treat everyone equally, regardless of background.
- Dependability and Safety: AI should be reliable and safe to build trust.
- Transparency: Interpretability is crucial for AI to communicate effectively, making predictions clear.
- Privacy and Security: Secure configurations for AI should be developed to ensure data transparency and responsible handling, thereby keeping data safe and maintaining customers’ trust
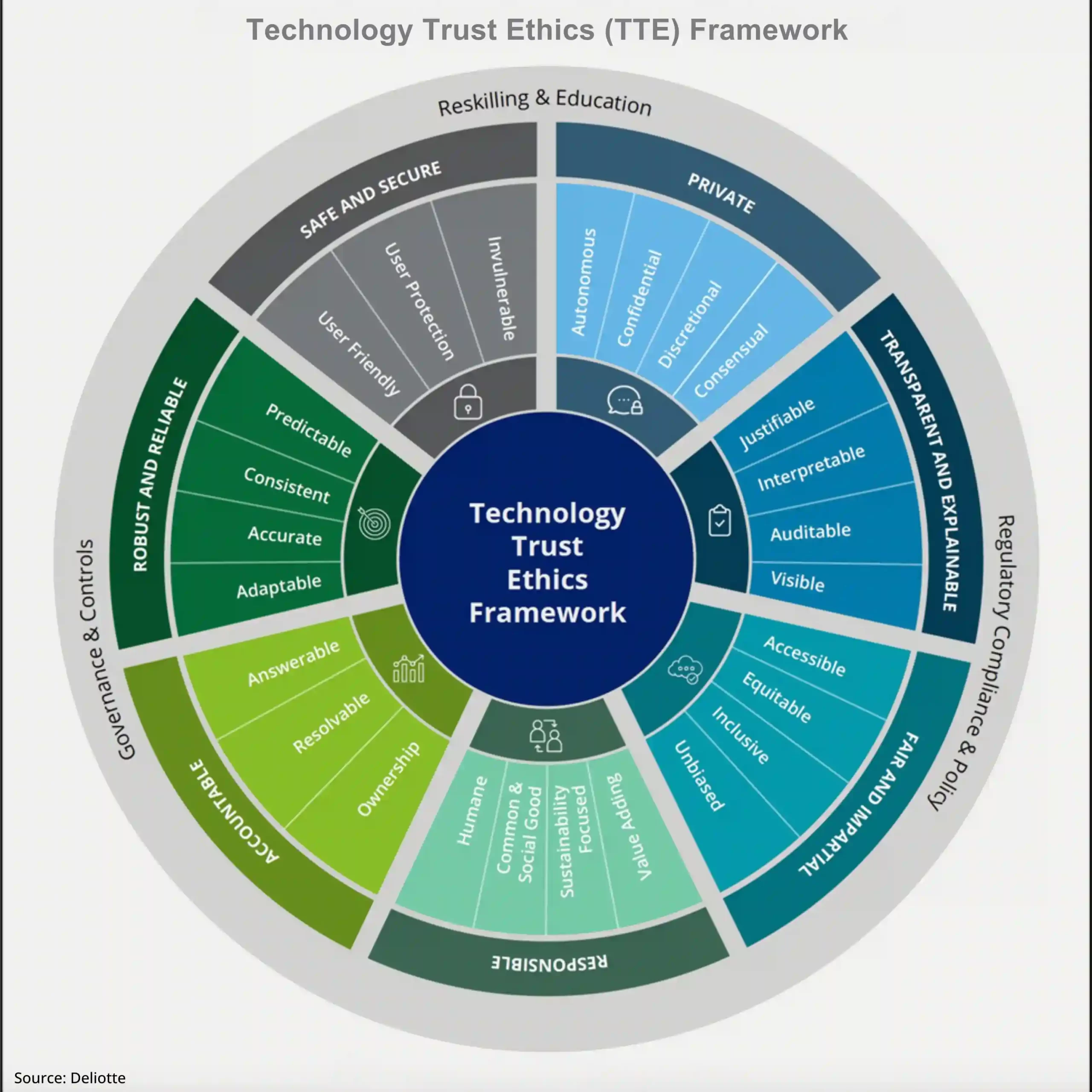
Strategies for Identifying and Addressing Bias in AI Systems
• Diverse Data Collection: Training data should reflect the diverse population to provide equitable decisions.
• Algorithmic Auditing: Rough testing and evaluation can reveal and quantify biases in AI systems.
• Interdisciplinary Collaborations: Collaboration between AI researchers and domain specialists can reveal potential biases.
• Transparency and Explainability: Understanding AI models’ decision-making processes can assist in detecting sources of bias and implementing remedial actions.
The growing usage of generative AI technologies requires a shift in decision-making transparency and explainability, as algorithms frequently operate as “black boxes.”
Transparency allows for research and validation, which promotes fairness and identifies any biases. Explainability boosts user trust, making AI technology more widely adopted. Balancing transparency and privacy protection is tough. However, regulatory agencies are developing guidelines to encourage AI developers to prioritize these concerns.
AI’s Impact on B2B Operations
AI is transforming B2B operations by increasing efficiency and customer satisfaction; nevertheless, responsible application necessitates transparency, data security, privacy, fairness, governance, and trust. Fairness and prejudice mitigation are critical, but human monitoring must be maintained. Governance and accountability are critical, with a dedicated AI governance body and frequent audits. Human-AI collaboration and customer trust are also principal factors to consider. AI in B2B can boost lead generation and cold calling.
Benefits of Responsible AI Development for Businesses
Responsible AI is important for businesses to develop, remain competitive, and reduce risks. It integrates AI resources with company values, lowering the risk of failure and harm to individuals and society. Microsoft and IBM have established responsible AI governance frameworks to promote inclusive governance and employee empowerment. Responsible AI, such as IBM Watson Health, supports healthcare diagnosis by analyzing large amounts of medical data, increasing early illness identification, and protecting personal privacy, resulting in better patient outcomes.
Takeaways
• Responsible AI development prioritizes safety, trustworthiness, and ethics.
• Biased AI systems may favor certain groups over others, resulting in inaccurate ratings.
• Biased predictions can hurt crucial fields such as healthcare and finance, undermining public trust in artificial intelligence.
• Creating trustworthy AI requires fairness, inclusivity, dependability, safety, transparency, privacy, and security.
• Strategies for bias reduction include diversified data gathering, algorithmic audits, interdisciplinary cooperation, and transparency.
Conclusion
AI’s evolution presents both challenges and opportunities. To eliminate bias, transparency, ethics, data privacy, and responsible AI integration, it is crucial to employ techniques like regularization and re-weighting, algorithmic fairness methods, and regular audits. AI bias can manifest in biased language, discriminatory medical diagnoses, or skewed criminal sentencing predictions. Three sources of bias in AI are data bias, algorithmic bias, and user-generated bias.
Enroll in the AI+ Engineer™ certification, which offers a systematic approach encompassing AI principles, techniques, and practical applications, with a focus on responsible AI development.
You may also want to read more on “Building Trust in AI: Unlocking Transparency and Employee Advocacy.”